Unlock the Power of Convenience: Discover Your Ultimate Handheld Air Compressor Today!
Handheld air compressors are becoming increasingly popular among DIY enthusiasts and automotive aficionados alike. These compact devices offer a level of convenience that makes everyday tasks, such as inflating tires or sports equipment, quick and hassle-free. Imagine being able to pump up a flat tire in mere minutes or inflate a pool float with ease during a sunny day—this is the power of a handheld air compressor. With a variety of models available, each tailored for specific needs, choosing the right compressor can significantly enhance your efficiency and productivity. In this article, we’ll explore what handheld air compressors are, their benefits, features to consider when purchasing, common uses, and maintenance tips to ensure your compressor serves you well for years to come.
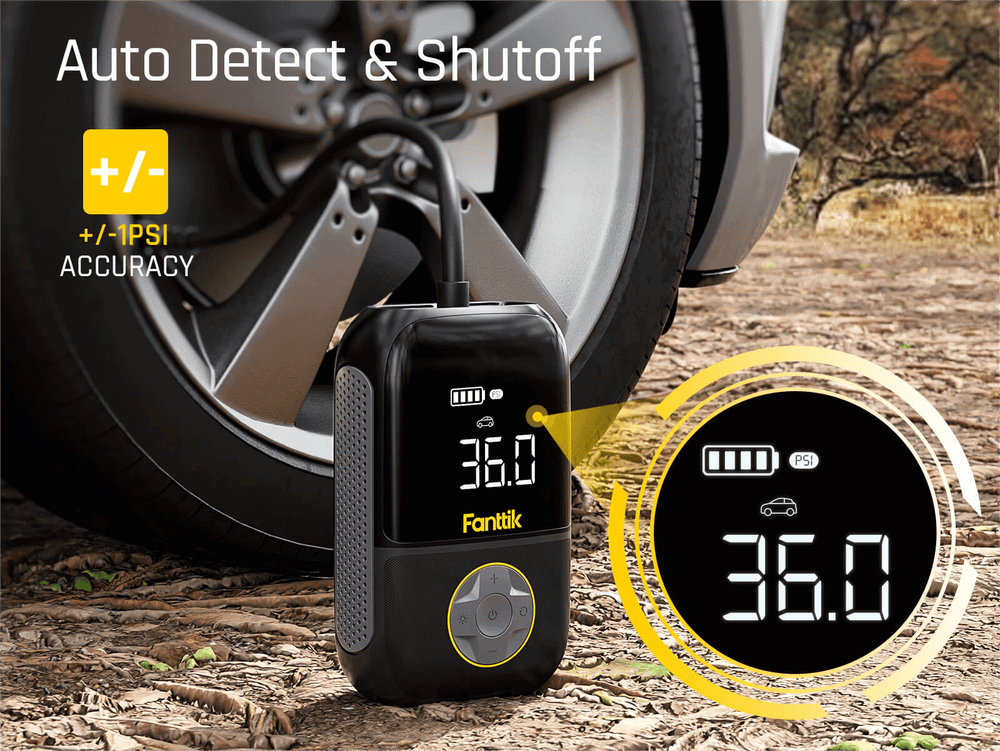
Understanding Handheld Air Compressors
Handheld air compressors are portable devices designed to generate compressed air, making them perfect for a variety of tasks. These compressors typically operate using either a rechargeable battery or a power cord that plugs into an outlet. Most handheld models are lightweight and compact, allowing for easy transportation and storage. Key features include a pressure gauge to monitor air pressure, a hose for directing air, and various nozzles for different applications. The pressure capabilities of these compressors can vary widely, so it’s important to choose one that matches your intended use—whether it’s inflating a tire or powering pneumatic tools. Understanding how these devices work can help you make an informed decision when selecting a model that suits your needs.
Benefits of Using a Handheld Air Compressor
The advantages of owning a handheld air compressor are numerous. For automotive applications, they allow for quick tire inflation, which can be a lifesaver in emergencies. Additionally, these compressors can be used for household tasks, such as inflating air mattresses or cleaning hard-to-reach areas with compressed air. The versatility doesn’t end there; recreational activities like sports also benefit from having a handheld compressor for inflating balls and other equipment. Beyond convenience, these compressors save time and effort, allowing you to get back to what you love doing without the hassle of manual inflation. Personal experiences from friends highlight how a handheld compressor can turn a tedious job into a simple task, making it an invaluable tool in their arsenal.
Key Features to Consider When Purchasing
When selecting a handheld air compressor, several features should be evaluated to ensure you get the best model for your needs. First, consider the size and weight of the compressor; a lightweight and compact design is easier to handle and store. Next, look at the air pressure capacity, as different tasks require different levels of pressure. Battery life is another crucial factor, especially for those who plan to use their compressor frequently away from a power source. Additionally, consider any included accessories such as different nozzle attachments and hoses, which can enhance the compressor's versatility. Ultimately, understanding your intended use will guide you in prioritizing these features when making a purchase.
Common Uses for Handheld Air Compressors
Handheld air compressors are incredibly versatile, finding a place in numerous everyday applications. One of the primary uses is inflating car tires, which can be done quickly, ensuring that you always drive safely. Beyond vehicles, these compressors are perfect for inflating sports equipment like basketballs, soccer balls, and bike tires, making them essential for athletes and outdoor enthusiasts. They also come in handy for inflating air mattresses, ensuring your guests are comfortable without the hassle of manual pumping. Furthermore, handheld air compressors can assist in DIY projects, offering the capability to clean tools and surfaces with compressed air. The adaptability of these devices reflects their importance in both personal and professional settings.
Tips for Maintaining Your Handheld Air Compressor
Additionally, after using your handheld air compressor, it’s essential to conduct checks to ensure its optimal performance. Regular maintenance can significantly extend the life of the tool. To prevent rust and deterioration, store your compressor in a dry place and keep it free from dust and debris. Each time you use it, wipe down the exterior and check for signs of wear or buildup, especially in hoses and filters. A little maintenance goes a long way in ensuring that your handheld air compressor remains reliable for years to come.
Unlocking the Full Potential of Handheld Air Compressors
In conclusion, handheld air compressors are invaluable tools that offer convenience and versatility for both personal and automotive uses. From quickly inflating tires to assisting with household chores and recreational activities, their benefits are undeniable. As you consider purchasing a handheld air compressor, think about your specific needs and the features that will best serve you. With the right model in hand, you'll unlock a new level of convenience that can simplify your daily tasks and enhance your overall productivity.